Case
What is the fastest way to using Extract Load Transform (ELT) on a Massively Parallel Processing (MPP) system like on a Azure SQL Datawarehouse.
![]() |
CTAS: The way to go! |
Solution
When Extracting, Loading and Transforming data on a MPP system like Azure SQL Data Warehouse there are several ways to do just that. Currently Create Table As Select (CTAS) is by far the fastest.Whats is a MPP system?
MPP stands for Massively Parallel Processing and is a database system that uses a control node to distribute the data across several seperate Compute Nodes. This makes it possible to load very large amounts of data in a fast way. All this is done automatically and for the end-user it appears to be one database. When you use traditional ETL software like SSIS to extract, load and transform data you make use of the memory that is allocated to the SSIS system and thus take the data out of the database, which is far less efficient.
![]() |
How a MPP looks like under the hood |
1) What is CTAS?
CTAS stands for Create Table As Select. As the name suggests the operation creates a new table using a select statement and is super fast. CTAS is fast because the data stays on the MPP and thus makes use of all the capabilities of a MPP system.
2) How to use CTAS
When you create a CTAS statement, you can choose to set two options, namely;
- Distribution options - Setting this option is mandatory
- Table options - Setting this option is optional. When not supplied a Clustered ColumnStore Index is used.
Distribution options
When you create the CTAS command you can choose between HASH, ROUND ROBIN or Replicate as distribution option.HASH is used to divide the data in equal sized sections and distribute them to the nodes using a distribution column. When doing this you try to evenly distribute the data on the available nodes. Choosing the correct distribution key here is paramount otherwise you can get skew on the distribution between the nodes. To put it simply; when you have 4 nodes and the data is not distributed evenly then it hurts the data retrieval speed. For example you want to use this option when creating Fact tables that are large (or very large Dimension tables). You can check for skew using the command
DBCC PDW_SHOWSPACEUSED
--Creating a table using CTAS and Hash CREATE TABLE dbo.CTASHash WITH ( DISTRIBUTION = HASH(FactCallCenterID) ) AS SELECT FactCallCenterID , DateKey , WageType , Calls , AutomaticResponses , Orders , IssuesRaised , AverageTimePerIssue , ServiceGrade FROM dbo.FactCallCenter
ROUND ROBIN is used when you dont want to choose a distribution column but are fine when the data is distributed randomly across the nodes. This is also the default option when you do not define a distribution option. This option is used for example for Staging tables. It is advised to always explicitly define the ROUND ROBIN in the CTAS statement.
--Creating a table using CTAS and Round Robin CREATE TABLE dbo.CTASRobin WITH ( DISTRIBUTION = ROUND_ROBIN ) AS SELECT AccountKey , ParentAccountKey , AccountCodeAlternateKey , ParentAccountCodeAlternateKey , ValueType , CustomMemberOptions FROM dbo.DimAccount
REPLICATE is used to put the data on every node available so it can be used for quick access. This is useful when creating regular sized Dimensions. When the data is available on each and every node then it safes on moving the data between nodes when using joins. The full table will be available on all nodes.
--Creating a table using CTAS and Replicate CREATE TABLE dbo.CTASReplicate WITH ( DISTRIBUTION = REPLICATE ) AS SELECT ScenarioKey , ScenarioName FROM dbo.DimScenario
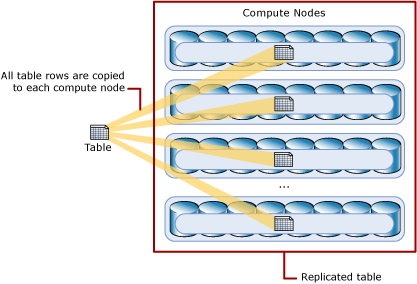
Table options
Besides choosing the distribution option, you can optionally also use the following table options;CLUSTERED COLUMNSTORE INDEX is a table option that is one of the most efficient ways to store data in Azure DWH. It improves data compression and query performance for data warehousing workloads and outperform Clustered Index and Heap tables. That makes them the best choice for large tables. Using a Clustered Columnstore Index is considered to be the best choice when you are unsure which table option you should best use. It is also the default table option when you only use one of the distribution options.
--Creating a table using CTAS and Clustered Columnstore Index CREATE TABLE dbo.CTASRobinCluster WITH ( DISTRIBUTION = ROUND_ROBIN , CLUSTERED COLUMNSTORE INDEX ) AS SELECT AccountKey , ParentAccountKey , AccountCodeAlternateKey , ParentAccountCodeAlternateKey , ValueType , CustomMemberOptions FROM dbo.DimAccount
HEAP is a table option that is usefull when temporarily loading data on Azure DWH and is the fastest way to load your data into a table. It is not advisable to use a heap table when the data in the table is frequently grouped together. That is because the data must be sorted before it can be grouped.
--Creating a table using CTAS and Heap CREATE TABLE dbo.CTASRobinHEAP WITH ( DISTRIBUTION = ROUND_ROBIN , HEAP) AS SELECT AccountKey , ParentAccountKey , AccountCodeAlternateKey , ParentAccountCodeAlternateKey , ValueType , CustomMemberOptions FROM dbo.DimAccount
CLUSTERED INDEX is a table option that you use when you want to sort and store the data rows in the table based on a specific column. The disadvantage of using a Clusted Index table is that only queries that use the defined Clustered Index column benefit from the index. This can be somewhat fixed by using additional Nonclustered indices, but that would increase use of space and processing time.
--Creating a table using CTAS and Clustered Index CREATE TABLE dbo.CTASRobinClusteredIndex WITH ( DISTRIBUTION = ROUND_ROBIN, CLUSTERED INDEX (AccountKey) ) AS SELECT AccountKey , ParentAccountKey , AccountCodeAlternateKey , ParentAccountCodeAlternateKey , ValueType , CustomMemberOptions FROM dbo.DimAccount
PARTITION is the table option that you use when you want to determine how the rows are grouped within each distribution. To use it you need to choose a partition column name. This column can be of any data type. You use partitioning to improve query performance and data maintenance and it avoids transaction logging. Using partitioning during the load proces can also substantially improve performance.
--Creating a table using CTAS and Partition CREATE TABLE dbo.CTASRobinPartition WITH ( DISTRIBUTION = HASH(ProductKey), CLUSTERED COLUMNSTORE INDEX, PARTITION ( OrderDateKey RANGE RIGHT FOR VALUES ( 20000101,20010101,20020101,20030101,20040101,20050101,20060101,20070101,20080101,20090101, 20100101,20110101,20120101,20130101,20140101,20150101,20160101,20170101,20180101,20190101, 20200101,20210101,20220101,20230101,20240101,20250101,20260101,20270101,20280101,20290101 ) ) ) AS SELECT ProductKey ,OrderDateKey ,DueDateKey ,ShipDateKey ,SalesAmount ,TaxAmt FROM dbo.FactInternetSales
3) Advantages of CTAS
With CTAS you are able to create and recreate tables using a specific distribution type and its very fast. If you have created HEAP tables and want to see if a different distribution type is a better option, then you can simply recreate the table using CTAS with the desired Distribution option. Simply create a copy of the table with a different name, drop the old table and rename the copy table to the original table name.
--Creating a table using CTAS and Partition CREATE TABLE dbo.CTASRenameTemp WITH ( DISTRIBUTION = ROUND_ROBIN ) AS SELECT * FROM dbo.DimAccount; DROP TABLE dbo.CTASRename; RENAME OBJECT dbo.CTASRenameTempTO CTASRename;
3) CTAS tips
When you create a copy a table using CTAS and do not manipulate any of the columns, all the settings of the columns are left intact. For example the datatype and nullability. When you (re)create a column in the table then you explicitly have to cast the table and optionally use the ISNULL() function to set the nullability of the column. For the latter if you do not use the ISNULL() function then the column automatically is created allowing NULL. In below example the first column allows NULL's and the second doesnt.
--CTAS Tips CREATE TABLE dbo.CTASCasting WITH ( DISTRIBUTION = ROUND_ROBIN ) AS SELECT CAST(ValueType AS VARCHAR(100)) AS ValueTypeNull , ISNULL(CAST(ValueType AS VARCHAR(100)),'Do not allow NULL') AS ValueTypeNotNull FROM dbo.DimAccount;